This is the multi-page printable view of this section. Click here to print.
Applications
1 - Specifying a Disruption Budget for Kubernetes Controllers
Introduction of Disruptions
We need to understand that some kind of voluntary disruptions can happen to pods. For example, they can be caused by cluster administrators who want to perform automated cluster actions, like upgrading and autoscaling clusters. Typical application owner actions include:
- deleting the deployment or other controller that manages the pod
- updating a deployment’s pod template causing a restart
- directly deleting a pod (e.g., by accident)
Setup Pod Disruption Budgets
Kubernetes offers a feature called PodDisruptionBudget (PDB) for each application. A PDB limits the number of pods of a replicated application that are down simultaneously from voluntary disruptions.
The most common use case is when you want to protect an application specified by one of the built-in Kubernetes controllers:
- Deployment
- ReplicationController
- ReplicaSet
- StatefulSet
A PodDisruptionBudget has three fields:
- A label selector
.spec.selector
to specify the set of pods to which it applies. .spec.minAvailable
which is a description of the number of pods from that set that must still be available after the eviction, even in the absence of the evicted pod. minAvailable can be either an absolute number or a percentage..spec.maxUnavailable
which is a description of the number of pods from that set that can be unavailable after the eviction. It can be either an absolute number or a percentage.
Cluster Upgrade or Node Deletion Failed due to PDB Violation:
Misconfiguration of the PDB could block the cluster upgrade or node deletion processes. There are two main cases that can cause a misconfiguration.
Case 1: The replica of Kubernetes controllers is 1
- Only 1 replica is running: there is no
replicaCount
setup orreplicaCount
for the Kubernetes controllers is set to 1 - PDB configuration
spec: minAvailable: 1
- To fix this PDB misconfiguration, you need to change the value of
replicaCount
for the Kubernetes controllers to a number greater than 1
Case 2: HPA configuration violates PDB
In Kubernetes, a HorizontalPodAutoscaler automatically updates a workload resource (such as a Deployment or StatefulSet), with the aim of automatically scaling the workload to match demand. The HorizontalPodAutoscaler manages the replicas field of the Kubernetes controllers.
- There is no
replicaCount
setup orreplicaCount
for the Kubernetes controllers is set to 1 - PDB configuration
spec: minAvailable: 1
- HPA configuration
spec: minReplicas: 1
- To fix this PDB misconfiguration, you need to change the value of HPA
minReplicas
to be greater than 1
Related Links
2 - Access a Port of a Pod Locally
Question
You have deployed an application with a web UI or an internal endpoint in your Kubernetes (K8s) cluster. How to access this endpoint without an external load balancer (e.g. Ingress)?
This tutorial presents two options:
- Using Kubernetes port forward
- Using Kubernetes apiserver proxy
Please note that the options described here are mostly for quick testing or troubleshooting your application. For enabling access to your application for productive environment, please refer to the official Kubernetes documentation.
Solution 1: Using Kubernetes port forward
You could use the port forwarding functionality of kubectl
to access the pods from your local host without involving a service.
To access any pod follow these steps:
- Run
kubectl get pods
- Note down the name of the pod in question as
<your-pod-name>
- Run
kubectl port-forward <your-pod-name> <local-port>:<your-app-port>
- Run a web browser or curl locally and enter the URL:
http(s)://localhost:<local-port>
In addition, kubectl port-forward
allows using a resource name, such as a deployment name or service name, to select a matching pod to port forward.
More details can be found in the Kubernetes documentation.
The main drawback of this approach is that the pod’s name changes as soon as it is restarted. Moreover, you need to have a web browser on your client and you need to make sure that the local port is not already used by an application running on your system. Finally, sometimes the port forwarding is canceled due to nonobvious reasons. This leads to a kind of shaky approach. A more stable possibility is based on accessing the app via the kube-proxy, which accesses the corresponding service.
Solution 2: Using the apiserver proxy of Your Kubernetes Cluster
There are several different proxies in Kubernetes. In this tutorial we will be using apiserver proxy to enable the access to the services in your cluster without Ingress. Unlike the first solution, here a service is required.
Use the following format to compose a URL for accessing your service through an existing proxy on the Kubernetes cluster:
https://<your-cluster-master>/api/v1/namespace/<your-namespace>/services/<your-service>:<your-service-port>/proxy/<service-endpoint>
Example:
your-main-cluster | your-namespace | your-service | your-service-port | your-service-endpoint | url to access service |
---|---|---|---|---|---|
api.testclstr.cpet.k8s.sapcloud.io | default | nginx-svc | 80 | / | http://api.testclstr.cpet.k8s.sapcloud.io/api/v1/namespaces/default/services/nginx-svc:80/proxy/ |
api.testclstr.cpet.k8s.sapcloud.io | default | docker-nodejs-svc | 4500 | /cpu?baseNumber=4 | https://api.testclstr.cpet.k8s.sapcloud.io/api/v1/namespaces/default/services/docker-nodejs-svc:4500/proxy/cpu?baseNumber=4 |
For more details on the format, please refer to the official Kubernetes documentation.
Note
There are applications which do not support relative URLs yet, e.g. Prometheus (as of November, 2022). This typically leads to missing JavaScript objects, which could be investigated with your browser’s development tools. If such an issue occurs, please use theport-forward
approach described above.3 - Auditing Kubernetes for Secure Setup
Increasing the Security of All Gardener Stakeholders
In summer 2018, the Gardener project team asked Kinvolk to execute several penetration tests in its role as third-party contractor. The goal of this ongoing work was to increase the security of all Gardener stakeholders in the open source community. Following the Gardener architecture, the control plane of a Gardener managed shoot cluster resides in the corresponding seed cluster. This is a Control-Plane-as-a-Service with a network air gap.
Along the way we found various kinds of security issues, for example, due to misconfiguration or missing isolation, as well as two special problems with upstream Kubernetes and its Control-Plane-as-a-Service architecture.
Major Findings
From this experience, we’d like to share a few examples of security issues that could happen on a Kubernetes installation and how to fix them.
Alban Crequy (Kinvolk) and Dirk Marwinski (SAP SE) gave a presentation entitled Hardening Multi-Cloud Kubernetes Clusters as a Service at KubeCon 2018 in Shanghai presenting some of the findings.
Here is a summary of the findings:
Privilege escalation due to insecure configuration of the Kubernetes API server
- Root cause: Same certificate authority (CA) is used for both the API server and the proxy that allows accessing the API server.
- Risk: Users can get access to the API server.
- Recommendation: Always use different CAs.
Exploration of the control plane network with malicious HTTP-redirects
Root cause: See detailed description below.
Risk: Provoked error message contains full HTTP payload from an existing endpoint which can be exploited. The contents of the payload depends on your setup, but can potentially be user data, configuration data, and credentials.
Recommendation:
- Use the latest version of Gardener
- Ensure the seed cluster’s container network supports network policies. Clusters that have been created with Kubify are not protected as Flannel is used there which doesn’t support network policies.
Reading private AWS metadata via Grafana
- Root cause: It is possible to configuring a new custom data source in Grafana, we could send HTTP requests to target the control
- Risk: Users can get the “user-data” for the seed cluster from the metadata service and retrieve a kubeconfig for that Kubernetes cluster
- Recommendation: Lockdown Grafana features to only what’s necessary in this setup, block all unnecessary outgoing traffic, move Grafana to a different network, lockdown unauthenticated endpoints
Scenario 1: Privilege Escalation with Insecure API Server
In most configurations, different components connect directly to the Kubernetes API server, often using a kubeconfig
with a client
certificate. The API server is started with the flag:
/hyperkube apiserver --client-ca-file=/srv/kubernetes/ca/ca.crt ...
The API server will check whether the client certificate presented by kubectl, kubelet, scheduler or another component is really signed by the configured certificate authority for clients.
The API server can have many clients of various kinds
However, it is possible to configure the API server differently for use with an intermediate authenticating proxy. The proxy will authenticate the client with its own custom method and then issue HTTP requests to the API server with additional HTTP headers specifying the user name and group name. The API server should only accept HTTP requests with HTTP headers from a legitimate proxy. To allow the API server to check incoming requests, you need pass on a list of certificate authorities (CAs) to it. Requests coming from a proxy are only accepted if they use a client certificate that is signed by one of the CAs of that list.
--requestheader-client-ca-file=/srv/kubernetes/ca/ca-proxy.crt
--requestheader-username-headers=X-Remote-User
--requestheader-group-headers=X-Remote-Group
API server clients can reach the API server through an authenticating proxy
So far, so good. But what happens if the malicious user “Mallory” tries to connect directly to the API server and reuses the HTTP headers to pretend to be someone else?
What happens when a client bypasses the proxy, connecting directly to the API server?
With a correct configuration, Mallory’s kubeconfig will have a certificate signed by the API server certificate authority but not signed by the proxy certificate authority. So the API server will not accept the extra HTTP header “X-Remote-Group: system:masters”.
You only run into an issue when the same certificate authority is used for both the API server and the proxy. Then, any Kubernetes client certificate can be used to take the role of different user or group as the API server will accept the user header and group header.
The kubectl
tool does not normally add those HTTP headers but it’s pretty easy to generate the corresponding HTTP
requests manually.
We worked on improving the Kubernetes documentation to make clearer that this configuration should be avoided.
Scenario 2: Exploration of the Control Plane Network with Malicious HTTP-Redirects
The API server is a central component of Kubernetes and many components initiate connections to it, including the kubelet running on worker nodes. Most of the requests from those clients will end up updating Kubernetes objects (pods, services, deployments, and so on) in the etcd database but the API server usually does not need to initiate TCP connections itself.
The API server is mostly a component that receives requests
However, there are exceptions. Some kubectl
commands will trigger the API server to open a new
connection to the kubelet. kubectl exec
is one of those commands. In order to get the standard I/Os from the pod,
the API server will start an HTTP connection to the kubelet on the worker node where the pod is running. Depending on
the container runtime used, it can be done in different ways, but one way to do it is for the kubelet to reply with a
HTTP-302 redirection to the Container Runtime Interface (CRI).
Basically, the kubelet is telling the API server to get the streams from CRI itself directly instead of forwarding. The
redirection from the kubelet will only change the port and path from the URL; the IP address will not be changed because
the kubelet and the CRI component run on the same worker node.
But the API server also initiates some connections, for example, to worker nodes
It’s often quite easy for users of a Kubernetes cluster to get access to worker nodes and tamper with the kubelet. They could be given explicit SSH access or they could be given a kubeconfig with enough privileges to create privileged pods or even just pods with “host” volumes.
In contrast, users (even those with “system:masters” permissions or “root” rights) are often not given access to the control plane. On setups like, for example, GKE or Gardener, the control plane is running on separate nodes, with a different administrative access. It could be hosted on a different cloud provider account. So users are not free to explore the internal network in the control plane.
What would happen if a user was tampering with the kubelet to make it maliciously redirect kubectl exec
requests to
a different random endpoint? Most likely the given endpoint would not speak to the streaming server protocol, so there would
be an error. However, the full HTTP payload from the endpoint is included in the error message printed by kubectl exec.
The API server is tricked to connect to other components
The impact of this issue depends on the specific setup. But in many configurations, we could find a metadata service (such as the AWS metadata service) containing user data, configurations and credentials. The setup we explored had a different AWS account and a different EC2 instance profile for the worker nodes and the control plane. This issue allowed users to get access to the AWS metadata service in the context of the control plane, which they should not have access to.
We have reported this issue to the Kubernetes Security mailing list and the public pull request that addresses the issue has been merged PR#66516. It provides a way to enforce HTTP redirect validation (disabled by default).
But there are several other ways that users could trigger the API server to generate HTTP requests and get the reply payload back, so it is advised to isolate the API server and other components from the network as additional precautious measures. Depending on where the API server runs, it could be with Kubernetes Network Policies, EC2 Security Groups or just iptables directly. Following the defense in depth principle, it is a good idea to apply the API server HTTP redirect validation when it is available as well as firewall rules.
In Gardener, this has been fixed with Kubernetes network policies along with changes to ensure the API server does not need to contact the metadata service. You can see more details in the announcements on the Gardener mailing list. This is tracked in CVE-2018-2475.
To be protected from this issue, stakeholders should:
- Use the latest version of Gardener
- Ensure the seed cluster’s container network supports network policies. Clusters that have been created with Kubify are not protected as Flannel is used there which doesn’t support network policies.
Scenario 3: Reading Private AWS Metadata via Grafana
For our tests, we had access to a Kubernetes setup where users are not only given access to the API server in the control plane, but also to a Grafana instance that is used to gather data from their Kubernetes clusters via Prometheus. The control plane is managed and users don’t have access to the nodes that it runs. They can only access the API server and Grafana via a load balancer. The internal network of the control plane is therefore hidden to users.
Prometheus and Grafana can be used to monitor worker nodes
Unfortunately, that setup was not protecting the control plane network from nosy users. By configuring a new custom data source in Grafana, we could send HTTP requests to target the control plane network, for example the AWS metadata service. The reply payload is not displayed on the Grafana Web UI but it is possible to access it from the debugging console of the Chrome browser.
Credentials can be retrieved from the debugging console of Chrome
Adding a Grafana data source is a way to issue HTTP requests to arbitrary targets
In that installation, users could get the “user-data” for the seed cluster from the metadata service and retrieve a kubeconfig for that Kubernetes cluster.
There are many possible measures to avoid this situation: lockdown Grafana features to only what’s necessary in this setup, block all unnecessary outgoing traffic, move Grafana to a different network, or lockdown unauthenticated endpoints, among others.
Conclusion
The three scenarios above show pitfalls with a Kubernetes setup. A lot of them were specific to the Kubernetes installation: different cloud providers or different configurations will show different weaknesses. Users should no longer be given access to Grafana.
4 - Container Image Not Pulled
Problem
Two of the most common causes of this problems are specifying the wrong container image or trying to use private images without providing registry credentials.
Note
There is no observable difference in pod status between a missing image and incorrect registry permissions. In either case, Kubernetes will report anErrImagePull
status for the pods. For this reason, this article deals with
both scenarios.Example
Let’s see an example. We’ll create a pod named fail, referencing a non-existent Docker image:
kubectl run -i --tty fail --image=tutum/curl:1.123456
The command doesn’t return and you can terminate the process with Ctrl+C
.
Error Analysis
We can then inspect our pods and see that we have one pod with a status of ErrImagePull or ImagePullBackOff.
$ (minikube) kubectl get pods
NAME READY STATUS RESTARTS AGE
client-5b65b6c866-cs4ch 1/1 Running 1 1m
fail-6667d7685d-7v6w8 0/1 ErrImagePull 0 <invalid>
vuejs-578574b75f-5x98z 1/1 Running 0 1d
$ (minikube)
For some additional information, we can describe
the failing pod.
kubectl describe pod fail-6667d7685d-7v6w8
As you can see in the events section, your image can’t be pulled:
Name: fail-6667d7685d-7v6w8
Namespace: default
Node: minikube/192.168.64.10
Start Time: Wed, 22 Nov 2017 10:01:59 +0100
Labels: pod-template-hash=2223832418
run=fail
Annotations: kubernetes.io/created-by={"kind":"SerializedReference","apiVersion":"v1","reference":{"kind":"ReplicaSet","namespace":"default","name":"fail-6667d7685d","uid":"cc4ccb3f-cf63-11e7-afca-4a7a1fa05b3f","a...
.
.
.
.
Events:
FirstSeen LastSeen Count From SubObjectPath Type Reason Message
--------- -------- ----- ---- ------------- -------- ------ -------
1m 1m 1 default-scheduler Normal Scheduled Successfully assigned fail-6667d7685d-7v6w8 to minikube
1m 1m 1 kubelet, minikube Normal SuccessfulMountVolume MountVolume.SetUp succeeded for volume "default-token-9fr6r"
1m 6s 4 kubelet, minikube spec.containers{fail} Normal Pulling pulling image "tutum/curl:1.123456"
1m 5s 4 kubelet, minikube spec.containers{fail} Warning Failed Failed to pull image "tutum/curl:1.123456": rpc error: code = Unknown desc = Error response from daemon: manifest for tutum/curl:1.123456 not found
1m <invalid> 10 kubelet, minikube Warning FailedSync Error syncing pod
1m <invalid> 6 kubelet, minikube spec.containers{fail} Normal BackOff Back-off pulling image "tutum/curl:1.123456"
Why couldn’t Kubernetes pull the image? There are three primary candidates besides network connectivity issues:
- The image tag is incorrect
- The image doesn’t exist
- Kubernetes doesn’t have permissions to pull that image
If you don’t notice a typo in your image tag, then it’s time to test using your local machine. I usually start by
running docker pull on my local development machine with the exact same image tag. In this case, I would
run docker pull tutum/curl:1.123456
.
If this succeeds, then it probably means that Kubernetes doesn’t have the correct permissions to pull that image.
Add the docker registry user/pwd to your cluster:
kubectl create secret docker-registry dockersecret --docker-server=https://index.docker.io/v1/ --docker-username=<username> --docker-password=<password> --docker-email=<email>
If the exact image tag fails, then I will test without an explicit image tag:
docker pull tutum/curl
This command will attempt to pull the latest tag. If this succeeds, then that means the originally specified tag doesn’t exist. Go to the Docker registry and check which tags are available for this image.
If docker pull tutum/curl
(without an exact tag) fails, then we have a bigger problem - that image does not exist at all in our image registry.
5 - Container Image Not Updating
Introduction
A container image should use a fixed tag or the SHA of the image. It should not use the tags latest, head, canary, or other tags that are designed to be floating.
Problem
If you have encountered this issue, you have probably done something along the lines of:
- Deploy anything using an image tag (e.g.
cp-enablement/awesomeapp:1.0
) - Fix a bug in awesomeapp
- Build a new image and push it with the same tag (
cp-enablement/awesomeapp:1.0
) - Update the deployment
- Realize that the bug is still present
- Repeat steps 3-5 without any improvement
The problem relates to how Kubernetes decides whether to do a docker pull when starting a container.
Since we tagged our image as :1.0, the default pull policy is IfNotPresent. The Kubelet already has a local
copy of cp-enablement/awesomeapp:1.0
, so it doesn’t attempt to do a docker pull. When the new Pods come up,
they’re still using the old broken Docker image.
There are a couple of ways to resolve this, with the recommended one being to use unique tags.
Solution
In order to fix the problem, you can use the following bash script that runs anytime the deployment is updated to create a new tag and push it to the registry.
#!/usr/bin/env bash
# Set the docker image name and the corresponding repository
# Ensure that you change them in the deployment.yml as well.
# You must be logged in with docker login.
#
# CHANGE THIS TO YOUR Docker.io SETTINGS
#
PROJECT=awesomeapp
REPOSITORY=cp-enablement
# causes the shell to exit if any subcommand or pipeline returns a non-zero status.
#
set -e
# set debug mode
#
set -x
# build my nodeJS app
#
npm run build
# get the latest version ID from the Docker.io registry and increment them
#
VERSION=$(curl https://registry.hub.docker.com/v1/repositories/$REPOSITORY/$PROJECT/tags | sed -e 's/[][]//g' -e 's/"//g' -e 's/ //g' | tr '}' '\n' | awk -F: '{print $3}' | grep v| tail -n 1)
VERSION=${VERSION:1}
((VERSION++))
VERSION="v$VERSION"
# build the new docker image
#
echo '>>> Building new image'
echo '>>> Push new image'
docker push $REPOSITORY/$PROJECT:$VERSION
6 - Custom Seccomp Profile
Overview
Seccomp (secure computing mode) is a security facility in the Linux kernel for restricting the set of system calls applications can make.
Starting from Kubernetes v1.3.0, the Seccomp feature is in Alpha
. To configure it on a Pod
, the following annotations can be used:
seccomp.security.alpha.kubernetes.io/pod: <seccomp-profile>
where<seccomp-profile>
is the seccomp profile to apply to all containers in aPod
.container.seccomp.security.alpha.kubernetes.io/<container-name>: <seccomp-profile>
where<seccomp-profile>
is the seccomp profile to apply to<container-name>
in aPod
.
More details can be found in the PodSecurityPolicy
documentation.
Installation of a Custom Profile
By default, kubelet loads custom Seccomp profiles from /var/lib/kubelet/seccomp/
. There are two ways in which Seccomp profiles can be added to a Node
:
- to be baked in the machine image
- to be added at runtime
This guide focuses on creating those profiles via a DaemonSet
.
Create a file called seccomp-profile.yaml
with the following content:
apiVersion: v1
kind: ConfigMap
metadata:
name: seccomp-profile
namespace: kube-system
data:
my-profile.json: |
{
"defaultAction": "SCMP_ACT_ALLOW",
"syscalls": [
{
"name": "chmod",
"action": "SCMP_ACT_ERRNO"
}
]
}
Note
The policy above is a very simple one and not suitable for complex applications. The default docker profile can be used a reference. Feel free to modify it to your needs.Apply the ConfigMap
in your cluster:
$ kubectl apply -f seccomp-profile.yaml
configmap/seccomp-profile created
The next steps is to create the DaemonSet
Seccomp installer. It’s going to copy the policy from above in /var/lib/kubelet/seccomp/my-profile.json
.
Create a file called seccomp-installer.yaml
with the following content:
apiVersion: apps/v1
kind: DaemonSet
metadata:
name: seccomp
namespace: kube-system
labels:
security: seccomp
spec:
selector:
matchLabels:
security: seccomp
template:
metadata:
labels:
security: seccomp
spec:
initContainers:
- name: installer
image: alpine:3.10.0
command: ["/bin/sh", "-c", "cp -r -L /seccomp/*.json /host/seccomp/"]
volumeMounts:
- name: profiles
mountPath: /seccomp
- name: hostseccomp
mountPath: /host/seccomp
readOnly: false
containers:
- name: pause
image: k8s.gcr.io/pause:3.1
terminationGracePeriodSeconds: 5
volumes:
- name: hostseccomp
hostPath:
path: /var/lib/kubelet/seccomp
- name: profiles
configMap:
name: seccomp-profile
Create the installer and wait until it’s ready on all Nodes
:
$ kubectl apply -f seccomp-installer.yaml
daemonset.apps/seccomp-installer created
$ kubectl -n kube-system get pods -l security=seccomp
NAME READY STATUS RESTARTS AGE
seccomp-installer-wjbxq 1/1 Running 0 21s
Create a Pod Using a Custom Seccomp Profile
Finally, we want to create a profile which uses our new Seccomp profile my-profile.json
.
Create a file called my-seccomp-pod.yaml
with the following content:
apiVersion: v1
kind: Pod
metadata:
name: seccomp-app
namespace: default
annotations:
seccomp.security.alpha.kubernetes.io/pod: "localhost/my-profile.json"
# you can specify seccomp profile per container. If you add another profile you can configure
# it for a specific container - 'pause' in this case.
# container.seccomp.security.alpha.kubernetes.io/pause: "localhost/some-other-profile.json"
spec:
containers:
- name: pause
image: k8s.gcr.io/pause:3.1
Create the Pod
and see that it’s running:
$ kubectl apply -f my-seccomp-pod.yaml
pod/seccomp-app created
$ kubectl get pod seccomp-app
NAME READY STATUS RESTARTS AGE
seccomp-app 1/1 Running 0 42s
Throubleshooting
If an invalid or a non-existing profile is used, then the Pod
will be stuck in ContainerCreating
phase:
broken-seccomp-pod.yaml
:
apiVersion: v1
kind: Pod
metadata:
name: broken-seccomp
namespace: default
annotations:
seccomp.security.alpha.kubernetes.io/pod: "localhost/not-existing-profile.json"
spec:
containers:
- name: pause
image: k8s.gcr.io/pause:3.1
$ kubectl apply -f broken-seccomp-pod.yaml
pod/broken-seccomp created
$ kubectl get pod broken-seccomp
NAME READY STATUS RESTARTS AGE
broken-seccomp 1/1 ContainerCreating 0 2m
$ kubectl describe pod broken-seccomp
Name: broken-seccomp
Namespace: default
....
Events:
Type Reason Age From Message
---- ------ ---- ---- -------
Normal Scheduled 18s default-scheduler Successfully assigned kube-system/broken-seccomp to docker-desktop
Warning FailedCreatePodSandBox 4s (x2 over 18s) kubelet, docker-desktop Failed create pod sandbox: rpc error: code = Unknown desc = failed to make sandbox docker config for pod "broken-seccomp": failed to generate sandbox security options
for sandbox "broken-seccomp": failed to generate seccomp security options for container: cannot load seccomp profile "/var/lib/kubelet/seccomp/not-existing-profile.json": open /var/lib/kubelet/seccomp/not-existing-profile.json: no such file or directory
Related Links
7 - Dockerfile Pitfalls
Using the latest
Tag for an Image
Many Dockerfiles use the FROM package:latest
pattern at the top of their Dockerfiles to pull the latest image from a Docker registry.
Bad Dockerfile
FROM alpine
While simple, using the latest tag for an image means that your build can suddenly break if that image gets updated. This can lead to problems where everything builds fine locally (because your local cache thinks it is the latest), while a build server may fail, because some pipelines make a clean pull on every build. Additionally, troubleshooting can prove to be difficult, since the maintainer of the Dockerfile didn’t actually make any changes.
Good Dockerfile
A digest takes the place of the tag when pulling an image. This will ensure that your Dockerfile remains immutable.
FROM alpine@sha256:7043076348bf5040220df6ad703798fd8593a0918d06d3ce30c6c93be117e430
Running apt/apk/yum update
Running apt-get install
is one of those things virtually every Debian-based Dockerfile will have to do in order to satiate some external package requirements your code needs to run. However, using apt-get
as an example, this comes with its own problems.
apt-get upgrade
This will update all your packages to their latests versions, which can be bad because it prevents your Dockerfile from creating consistent, immutable builds.
apt-get update (in a different line than the one running your apt-get install command)
Running apt-get update
as a single line entry will get cached by the build and won’t actually run every time you need to run apt-get install
. Instead, make sure you run apt-get update
in the same line with all the packages to ensure that all are updated correctly.
Avoid Big Container Images
Building a small container image will reduce the time needed to start or restart pods. An image based on the popular Alpine Linux project is much smaller than most distribution based images (~5MB). For most popular languages and products, there is usually an official Alpine Linux image, e.g. golang, nodejs, and postgres.
$ docker images
REPOSITORY TAG IMAGE ID CREATED SIZE
postgres 9.6.9-alpine 6583932564f8 13 days ago 39.26 MB
postgres 9.6 d92dad241eff 13 days ago 235.4 MB
postgres 10.4-alpine 93797b0f31f4 13 days ago 39.56 MB
In addition, for compiled languages such as Go or C++ that do not require build time tooling during runtime, it is recommended to avoid build time tooling in the final images. With Docker’s support for multi-stages builds, this can be easily achieved with minimal effort. Such an example can be found at Multi-stage builds.
Google’s distroless image is also a good base image.
8 - Dynamic Volume Provisioning
Overview
The example shows how to run a Postgres database on Kubernetes and how to dynamically provision and mount the storage volumes needed by the database
Run Postgres Database
Define the following Kubernetes resources in a yaml file:
- PersistentVolumeClaim (PVC)
- Deployment
PersistentVolumeClaim
apiVersion: v1
kind: PersistentVolumeClaim
metadata:
name: postgresdb-pvc
spec:
accessModes:
- ReadWriteOnce
resources:
requests:
storage: 9Gi
storageClassName: 'default'
This defines a PVC using the storage class default
. Storage classes abstract from the underlying storage provider as well
as other parameters, like disk-type (e.g.; solid-state vs standard disks).
The default storage class has the annotation {“storageclass.kubernetes.io/is-default-class”:“true”}.
$ kubectl describe sc default
Name: default
IsDefaultClass: Yes
Annotations: kubectl.kubernetes.io/last-applied-configuration={"apiVersion":"storage.k8s.io/v1beta1","kind":"StorageClass","metadata":{"annotations":{"storageclass.kubernetes.io/is-default-class":"true"},"labels":{"addonmanager.kubernetes.io/mode":"Exists"},"name":"default","namespace":""},"parameters":{"type":"gp2"},"provisioner":"kubernetes.io/aws-ebs"}
,storageclass.kubernetes.io/is-default-class=true
Provisioner: kubernetes.io/aws-ebs
Parameters: type=gp2
AllowVolumeExpansion: <unset>
MountOptions: <none>
ReclaimPolicy: Delete
VolumeBindingMode: Immediate
Events: <none>
A Persistent Volume is automatically created when it is dynamically provisioned. In the following example, the PVC is defined as “postgresdb-pvc”, and a corresponding PV “pvc-06c81c30-72ea-11e8-ada2-aa3b2329c8bb” is created and associated with the PVC automatically.
$ kubectl create -f .\postgres_deployment.yaml
persistentvolumeclaim "postgresdb-pvc" created
$ kubectl get pv
NAME CAPACITY ACCESS MODES RECLAIM POLICY STATUS CLAIM STORAGECLASS REASON AGE
pvc-06c81c30-72ea-11e8-ada2-aa3b2329c8bb 9Gi RWO Delete Bound default/postgresdb-pvc default 3s
$ kubectl get pvc
NAME STATUS VOLUME CAPACITY ACCESS MODES STORAGECLASS AGE
postgresdb-pvc Bound pvc-06c81c30-72ea-11e8-ada2-aa3b2329c8bb 9Gi RWO default 8s
Notice that the RECLAIM POLICY is Delete (default value), which is one of the two reclaim policies, the other one is Retain. (A third policy Recycle has been deprecated). In the case of Delete, the PV is deleted automatically when the PVC is removed, and the data on the PVC will also be lost.
On the other hand, a PV with Retain policy will not be deleted when the PVC is removed, and moved to Release status, so that data can be recovered by Administrators later.
You can use the kubectl patch
command to change the reclaim policy as described in Change the Reclaim Policy of a PersistentVolume
or use kubectl edit pv <pv-name>
to edit it online as shown below:
$ kubectl get pv
NAME CAPACITY ACCESS MODES RECLAIM POLICY STATUS CLAIM STORAGECLASS REASON AGE
pvc-06c81c30-72ea-11e8-ada2-aa3b2329c8bb 9Gi RWO Delete Bound default/postgresdb-pvc default 44m
# change the reclaim policy from "Delete" to "Retain"
$ kubectl edit pv pvc-06c81c30-72ea-11e8-ada2-aa3b2329c8bb
persistentvolume "pvc-06c81c30-72ea-11e8-ada2-aa3b2329c8bb" edited
# check the reclaim policy afterwards
$ kubectl get pv
NAME CAPACITY ACCESS MODES RECLAIM POLICY STATUS CLAIM STORAGECLASS REASON AGE
pvc-06c81c30-72ea-11e8-ada2-aa3b2329c8bb 9Gi RWO Retain Bound default/postgresdb-pvc default 45m
Deployment
Once a PVC is created, you can use it in your container via volumes.persistentVolumeClaim.claimName
. In the below
example, the PVC postgresdb-pvc is mounted as readable and writable, and in volumeMounts
two paths in the container are mounted to subfolders in the volume.
apiVersion: apps/v1
kind: Deployment
metadata:
name: postgres
namespace: default
labels:
app: postgres
annotations:
deployment.kubernetes.io/revision: "1"
spec:
replicas: 1
strategy:
type: RollingUpdate
rollingUpdate:
maxUnavailable: 1
maxSurge: 1
selector:
matchLabels:
app: postgres
template:
metadata:
name: postgres
labels:
app: postgres
spec:
containers:
- name: postgres
image: "cpettech.docker.repositories.sap.ondemand.com/jtrack_postgres:howto"
env:
- name: POSTGRES_USER
value: postgres
- name: POSTGRES_PASSWORD
value: p5FVqfuJFrM42cVX9muQXxrC3r8S9yn0zqWnFR6xCoPqxqVQ
- name: POSTGRES_INITDB_XLOGDIR
value: "/var/log/postgresql/logs"
ports:
- containerPort: 5432
volumeMounts:
- mountPath: /var/lib/postgresql/data
name: postgre-db
subPath: data # https://github.com/kubernetes/website/pull/2292. Solve the issue of crashing initdb due to non-empty directory (i.e. lost+found)
- mountPath: /var/log/postgresql/logs
name: postgre-db
subPath: logs
volumes:
- name: postgre-db
persistentVolumeClaim:
claimName: postgresdb-pvc
readOnly: false
imagePullSecrets:
- name: cpettechregistry
To check the mount points in the container:
$ kubectl get po
NAME READY STATUS RESTARTS AGE
postgres-7f485fd768-c5jf9 1/1 Running 0 32m
$ kubectl exec -it postgres-7f485fd768-c5jf9 bash
root@postgres-7f485fd768-c5jf9:/# ls /var/lib/postgresql/data/
base pg_clog pg_dynshmem pg_ident.conf pg_multixact pg_replslot pg_snapshots pg_stat_tmp pg_tblspc PG_VERSION postgresql.auto.conf postmaster.opts
global pg_commit_ts pg_hba.conf pg_logical pg_notify pg_serial pg_stat pg_subtrans pg_twophase pg_xlog postgresql.conf postmaster.pid
root@postgres-7f485fd768-c5jf9:/# ls /var/log/postgresql/logs/
000000010000000000000001 archive_status
Deleting a PersistentVolumeClaim
In case of a Delete policy, deleting a PVC will also delete its associated PV. If Retain is the reclaim policy, the PV will change status from Bound to Released when the PVC is deleted.
# Check pvc and pv before deletion
$ kubectl get pvc
NAME STATUS VOLUME CAPACITY ACCESS MODES STORAGECLASS AGE
postgresdb-pvc Bound pvc-06c81c30-72ea-11e8-ada2-aa3b2329c8bb 9Gi RWO default 50m
$ kubectl get pv
NAME CAPACITY ACCESS MODES RECLAIM POLICY STATUS CLAIM STORAGECLASS REASON AGE
pvc-06c81c30-72ea-11e8-ada2-aa3b2329c8bb 9Gi RWO Retain Bound default/postgresdb-pvc default 50m
# delete pvc
$ kubectl delete pvc postgresdb-pvc
persistentvolumeclaim "postgresdb-pvc" deleted
# pv changed to status "Released"
$ kubectl get pv
NAME CAPACITY ACCESS MODES RECLAIM POLICY STATUS CLAIM STORAGECLASS REASON AGE
pvc-06c81c30-72ea-11e8-ada2-aa3b2329c8bb 9Gi RWO Retain Released default/postgresdb-pvc default 51m
9 - Install Knative in Gardener Clusters
Overview
This guide walks you through the installation of the latest version of Knative using pre-built images on a Gardener created cluster environment. To set up your own Gardener, see the documentation or have a look at the landscape-setup-template project. To learn more about this open source project, read the blog on kubernetes.io.
Prerequsites
Knative requires a Kubernetes cluster v1.15 or newer.
Steps
Install and Configure kubectl
If you already have
kubectl
CLI, runkubectl version --short
to check the version. You need v1.10 or newer. If yourkubectl
is older, follow the next step to install a newer version.
Access Gardener
Create a project in the Gardener dashboard. This will essentially create a Kubernetes namespace with the name
garden-<my-project>
.Configure access to your Gardener project using a kubeconfig.
If you are not the Gardener Administrator already, you can create a technical user in the Gardener dashboard. Go to the “Members” section and add a service account. You can then download the kubeconfig for your project. You can skip this step if you create your cluster using the user interface; it is only needed for programmatic access, make sure you set
export KUBECONFIG=garden-my-project.yaml
in your shell.
Creating a Kubernetes Cluster
You can create your cluster using kubectl
CLI by providing a cluster
specification yaml file. You can find an example for GCP in the
gardener/gardener repository.
Make sure the namespace matches that of your project. Then just apply the
prepared so-called “shoot” cluster CRD with kubectl:
kubectl apply --filename my-cluster.yaml
The easier alternative is to create the cluster following the cluster creation
wizard in the Gardener dashboard:
Configure kubectl for Your Cluster
You can now download the kubeconfig for your freshly created cluster in the Gardener dashboard or via the CLI as follows:
kubectl --namespace shoot--my-project--my-cluster get secret kubecfg --output jsonpath={.data.kubeconfig} | base64 --decode > my-cluster.yaml
This kubeconfig file has full administrators access to you cluster. For the rest
of this guide, be sure you have export KUBECONFIG=my-cluster.yaml
set.
Installing Istio
Knative depends on Istio. If your cloud platform offers a managed Istio installation, we recommend installing Istio that way, unless you need the ability to customize your installation.
Otherwise, see the Installing Istio for Knative guide to install Istio.
You must install Istio on your Kubernetes cluster before continuing with these instructions to install Knative.
Installing cluster-local-gateway
for Serving Cluster-Internal Traffic
If you installed Istio, you can install a cluster-local-gateway
within your Knative cluster so that you can serve cluster-internal traffic. If you want to configure your revisions to use routes that are visible only within your cluster, install and use the cluster-local-gateway
.
Installing Knative
The following commands install all available Knative components as well as the standard set of observability plugins. Knative’s installation guide - Installing Knative.
If you are upgrading from Knative 0.3.x: Update your domain and static IP address to be associated with the LoadBalancer
istio-ingressgateway
instead ofknative-ingressgateway
. Then run the following to clean up leftover resources:kubectl delete svc knative-ingressgateway -n istio-system kubectl delete deploy knative-ingressgateway -n istio-system
If you have the Knative Eventing Sources component installed, you will also need to delete the following resource before upgrading:
kubectl delete statefulset/controller-manager -n knative-sources
While the deletion of this resource during the upgrade process will not prevent modifications to Eventing Source resources, those changes will not be completed until the upgrade process finishes.
To install Knative, first install the CRDs by running the
kubectl apply
command once with the-l knative.dev/crd-install=true
flag. This prevents race conditions during the install, which cause intermittent errors:kubectl apply --selector knative.dev/crd-install=true \ --filename https://github.com/knative/serving/releases/download/v0.12.1/serving.yaml \ --filename https://github.com/knative/eventing/releases/download/v0.12.1/eventing.yaml \ --filename https://github.com/knative/serving/releases/download/v0.12.1/monitoring.yaml
To complete the installation of Knative and its dependencies, run the
kubectl apply
command again, this time without the--selector
flag:kubectl apply --filename https://github.com/knative/serving/releases/download/v0.12.1/serving.yaml \ --filename https://github.com/knative/eventing/releases/download/v0.12.1/eventing.yaml \ --filename https://github.com/knative/serving/releases/download/v0.12.1/monitoring.yaml
Monitor the Knative components until all of the components show a
STATUS
ofRunning
:kubectl get pods --namespace knative-serving kubectl get pods --namespace knative-eventing kubectl get pods --namespace knative-monitoring
Set Your Custom Domain
- Fetch the external IP or CNAME of the knative-ingressgateway:
kubectl --namespace istio-system get service knative-ingressgateway
NAME TYPE CLUSTER-IP EXTERNAL-IP PORT(S) AGE
knative-ingressgateway LoadBalancer 100.70.219.81 35.233.41.212 80:32380/TCP,443:32390/TCP,32400:32400/TCP 4d
- Create a wildcard DNS entry in your custom domain to point to the above IP or CNAME:
*.knative.<my domain> == A 35.233.41.212
# or CNAME if you are on AWS
*.knative.<my domain> == CNAME a317a278525d111e89f272a164fd35fb-1510370581.eu-central-1.elb.amazonaws.com
- Adapt your Knative config-domain (set your domain in the data field):
kubectl --namespace knative-serving get configmaps config-domain --output yaml
apiVersion: v1
data:
knative.<my domain>: ""
kind: ConfigMap
name: config-domain
namespace: knative-serving
What’s Next
Now that your cluster has Knative installed, you can see what Knative has to offer.
Deploy your first app with the Getting Started with Knative App Deployment guide.
Get started with Knative Eventing by walking through one of the Eventing Samples.
Install Cert-Manager if you want to use the automatic TLS cert provisioning feature.
Cleaning Up
Use the Gardener dashboard to delete your cluster, or execute the following with
kubectl pointing to your garden-my-project.yaml
kubeconfig:
kubectl --kubeconfig garden-my-project.yaml --namespace garden--my-project annotate shoot my-cluster confirmation.gardener.cloud/deletion=true
kubectl --kubeconfig garden-my-project.yaml --namespace garden--my-project delete shoot my-cluster
10 - Integrity and Immutability
Introduction
When transferring data among networked systems, trust is a central concern. In particular, when communicating over an untrusted medium such as the internet, it is critical to ensure the integrity and immutability of all the data a system operates on. Especially if you use Docker Engine to push and pull images (data) to a public registry.
This immutability offers you a guarantee that any and all containers that you instantiate will be absolutely identical at inception. Surprise surprise, deterministic operations.
A Lesson in Deterministic Ops
Docker Tags are about as reliable and disposable as this guy down here.
Seems simple enough. You have probably already deployed hundreds of YAML’s or started endless counts of Docker containers.
docker run --name mynginx1 -P -d nginx:1.13.9
or
apiVersion: apps/v1
kind: Deployment
metadata:
name: rss-site
spec:
replicas: 1
selector:
matchLabels:
app: web
template:
metadata:
labels:
app: web
spec:
containers:
- name: front-end
image: nginx:1.13.9
ports:
- containerPort: 80
But Tags are mutable and humans are prone to error. Not a good combination. Here, we’ll dig into why the use of tags can be dangerous and how to deploy your containers across a pipeline and across environments with determinism in mind.
Let’s say that you want to ensure that whether it’s today or 5 years from now, that specific deployment uses the very same image that you have defined. Any updates or newer versions of an image should be executed as a new deployment. The solution: digest
A digest takes the place of the tag when pulling an image. For example, to pull the above image by digest, run the following command:
docker run --name mynginx1 -P -d nginx@sha256:4771d09578c7c6a65299e110b3ee1c0a2592f5ea2618d23e4ffe7a4cab1ce5de
You can now make sure that the same image is always loaded at every deployment. It doesn’t matter if the TAG of the image has been changed or not. This solves the problem of repeatability.
Content Trust
However, there’s an additionally hidden danger. It is possible for an attacker to replace a server image with another one infected with malware.
Docker Content trust gives you the ability to verify both the integrity and the publisher of all the data received from a registry over any channel.
Prior to version 1.8, Docker didn’t have a way to verify the authenticity of a server image. But in v1.8, a new feature called Docker Content Trust was introduced to automatically sign and verify the signature of a publisher.
So, as soon as a server image is downloaded, it is cross-checked with the signature of the publisher to see if someone tampered with it in any way. This solves the problem of trust.
In addition, you should scan all images for known vulnerabilities.
11 - Kubernetes Antipatterns
This HowTo covers common Kubernetes antipatterns that we have seen over the past months.
Running as Root User
Whenever possible, do not run containers as root user. One could be tempted to say that Kubernetes pods and nodes are well separated. Host and containers running on it share the same kernel. If a container is compromised, the root user in the container has full control over the underlying node.
Watch the very good presentation by Liz Rice at the KubeCon 2018
Use RUN groupadd -r anygroup && useradd -r -g anygroup myuser
to create a group and add a user to it. Use the USER
command to switch to this user. Note that you may also consider to provide an explicit UID/GID if required.
For example:
ARG GF_UID="500"
ARG GF_GID="500"
# add group & user
RUN groupadd -r -g $GF_GID appgroup && \
useradd appuser -r -u $GF_UID -g appgroup
USER appuser
Store Data or Logs in Containers
Containers are ideal for stateless applications and should be transient. This means that no data or logs should be stored in the container, as they are lost when the container is closed. Use persistence volumes instead to persist data outside of containers. Using an ELK stack is another good option for storing and processing logs.
Using Pod IP Addresses
Each pod is assigned an IP address. It is necessary for pods to communicate with each other to build an application, e.g. an application must communicate with a database. Existing pods are terminated and new pods are constantly started. If you would rely on the IP address of a pod or container, you would need to update the application configuration constantly. This makes the application fragile.
Create services instead. They provide a logical name that can be assigned independently of the varying number and IP addresses of containers. Services are the basic concept for load balancing within Kubernetes.
More Than One Process in a Container
A docker file provides a CMD
and ENTRYPOINT
to start the image. CMD
is often used around a script that makes a configuration and then
starts the container. Do not try to start multiple processes with this script. It is important to consider the separation of concerns when creating docker images. Running multiple processes in a single pod makes managing your containers, collecting logs and updating each process more difficult.
You can split the image into multiple containers and manage them independently - even in one pod. Bear in mind that Kubernetes only monitors the process with PID=1
. If more than one process is started within a container, then these no longer fall under the control of Kubernetes.
Creating Images in a Running Container
A new image can be created with the docker commit
command. This is useful if changes have been made to the container and you want to persist them for later error analysis. However, images created like this are not reproducible and completely worthless for a CI/CD environment. Furthermore, another developer cannot recognize which components the image contains. Instead, always make changes to the docker file, close existing containers and start a new container with the updated image.
Saving Passwords in a docker Image 💀
Do not save passwords in a Docker file! They are in plain text and are checked into a repository. That makes them completely vulnerable even if you are using a private repository like the Artifactory.
Always use Secrets or ConfigMaps to provision passwords or inject them by mounting a persistent volume.
Using the ’latest’ Tag
Starting an image with tomcat is tempting. If no tags are specified, a container is started with the tomcat:latest
image. This image may no longer be up to date and refer to an older version instead. Running a production application requires complete control of the environment with exact versions of the image.
Make sure you always use a tag or even better the sha256 hash of the image e.g. tomcat@sha256:c34ce3c1fcc0c7431e1392cc3abd0dfe2192ffea1898d5250f199d3ac8d8720f
.
Why Use the sha256 Hash?
Tags are not immutable and can be overwritten by a developer at any time. In this case you don’t have complete control over your image - which is bad.
Different Images per Environment
Don’t create different images for development, testing, staging and production environments. The image should be the source of truth and should only be created once and pushed to the repository. This image:tag
should be used for different environments in the future.
Depend on Start Order of Pods
Applications often depend on containers being started in a certain order. For example, a database container must be up and running before an application can connect to it. The application should be resilient to such changes, as the db pod can be unreachable or restarted at any time. The application container should be able to handle such situations without terminating or crashing.
Additional Anti-Patterns and Patterns
In the community, vast experience has been collected to improve the stability and usability of Docker and Kubernetes.
Refer to Kubernetes Production Patterns for more information.
12 - Namespace Isolation
Overview
You can configure a NetworkPolicy to deny all the traffic from other namespaces while allowing all the traffic coming from the same namespace the pod was deployed into.
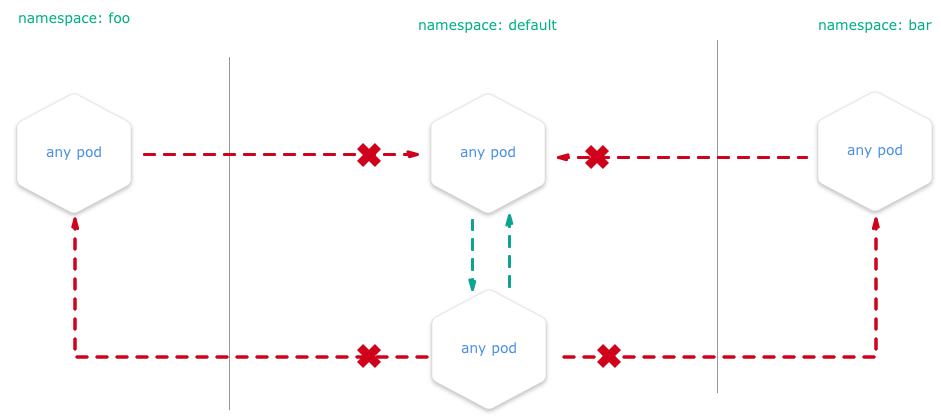
There are many reasons why you may chose to employ Kubernetes network policies:
- Isolate multi-tenant deployments
- Regulatory compliance
- Ensure containers assigned to different environments (e.g. dev/staging/prod) cannot interfere with each other
Kubernetes network policies are application centric compared to infrastructure/network centric standard firewalls. There are no explicit CIDRs or IP addresses used for matching source or destination IP’s. Network policies build up on labels and selectors which are key concepts of Kubernetes that are used to organize (for e.g all DB tier pods of an app) and select subsets of objects.
Example
We create two nginx HTTP-Servers in two namespaces and block all traffic between the two namespaces. E.g. you are unable to get content from namespace1 if you are sitting in namespace2.
Setup the Namespaces
# create two namespaces for test purpose
kubectl create ns customer1
kubectl create ns customer2
# create a standard HTTP web server
kubectl run nginx --image=nginx --replicas=1 --port=80 -n=customer1
kubectl run nginx --image=nginx --replicas=1 --port=80 -n=customer2
# expose the port 80 for external access
kubectl expose deployment nginx --port=80 --type=NodePort -n=customer1
kubectl expose deployment nginx --port=80 --type=NodePort -n=customer2
Test Without NP
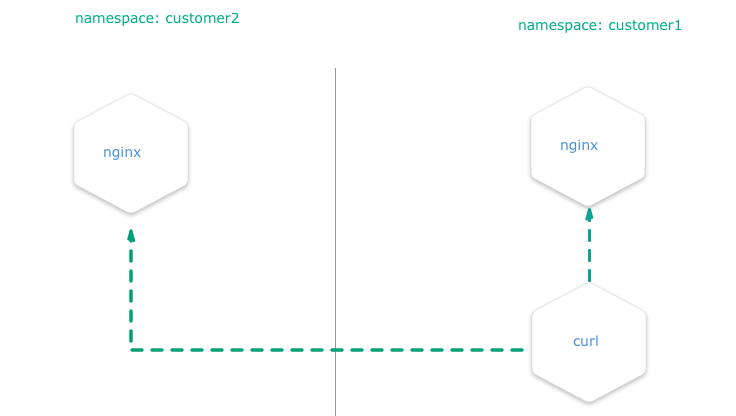
Create a pod with curl preinstalled inside the namespace customer1:
# create a "bash" pod in one namespace
kubectl run -i --tty client --image=tutum/curl -n=customer1
Try to curl the exposed nginx server to get the default index.html page. Execute this in the bash prompt of the pod created above.
# get the index.html from the nginx of the namespace "customer1" => success
curl http://nginx.customer1
# get the index.html from the nginx of the namespace "customer2" => success
curl http://nginx.customer2
Both calls are done in a pod within the namespace customer1 and both nginx servers are always reachable, no matter in what namespace.
Test with NP
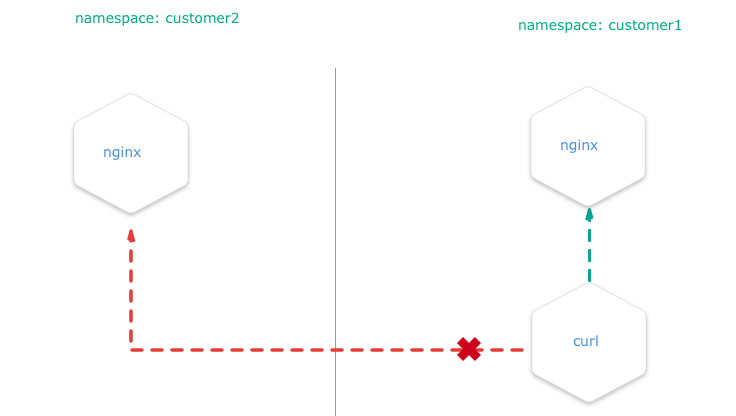
Install the NetworkPolicy from your shell:
apiVersion: networking.k8s.io/v1
kind: NetworkPolicy
metadata:
name: deny-from-other-namespaces
spec:
podSelector:
matchLabels:
ingress:
- from:
- podSelector: {}
- it applies the policy to ALL pods in the named namespace as the
spec.podSelector.matchLabels
is empty and therefore selects all pods. - it allows traffic from ALL pods in the named namespace, as
spec.ingress.from.podSelector
is empty and therefore selects all pods.
kubectl apply -f ./network-policy.yaml -n=customer1
kubectl apply -f ./network-policy.yaml -n=customer2
After this, curl http://nginx.customer2
shouldn’t work anymore if you are a service inside the namespace customer1 and
vice versa
Note
This policy, once applied, will also disable all external traffic to these pods. For example, you can create a service of typeLoadBalancer
in namespace customer1
that match the nginx pod. When you request the service by its <EXTERNAL_IP>:<PORT>
, then the network policy that will deny the ingress traffic from the service and the request will time out.Related Links
You can get more information on how to configure the NetworkPolicies at:
13 - Orchestration of Container Startup
Disclaimer
If an application depends on other services deployed separately, do not rely on a certain start sequence of containers. Instead, ensure that the application can cope with unavailability of the services it depends on.
Introduction
Kubernetes offers a feature called InitContainers
to perform some tasks during a pod’s initialization.
In this tutorial, we demonstrate how to use InitContainers
in order to orchestrate a starting sequence of multiple containers.
The tutorial uses the example app url-shortener,
which consists of two components:
- postgresql database
- webapp which depends on the postgresql database and provides two endpoints: create a short url from a given location and redirect from a given short URL to the corresponding target location
This app represents the minimal example where an application relies on another service or database. In this example, if the application starts before the database is ready, the application will fail as shown below:
$ kubectl logs webapp-958cf5567-h247n
time="2018-06-12T11:02:42Z" level=info msg="Connecting to Postgres database using: host=`postgres:5432` dbname=`url_shortener_db` username=`user`\n"
time="2018-06-12T11:02:42Z" level=fatal msg="failed to start: failed to open connection to database: dial tcp: lookup postgres on 100.64.0.10:53: no such host\n"
$ kubectl get po -w
NAME READY STATUS RESTARTS AGE
webapp-958cf5567-h247n 0/1 Pending 0 0s
webapp-958cf5567-h247n 0/1 Pending 0 0s
webapp-958cf5567-h247n 0/1 ContainerCreating 0 0s
webapp-958cf5567-h247n 0/1 ContainerCreating 0 1s
webapp-958cf5567-h247n 0/1 Error 0 2s
webapp-958cf5567-h247n 0/1 Error 1 3s
webapp-958cf5567-h247n 0/1 CrashLoopBackOff 1 4s
webapp-958cf5567-h247n 0/1 Error 2 18s
webapp-958cf5567-h247n 0/1 CrashLoopBackOff 2 29s
webapp-958cf5567-h247n 0/1 Error 3 43s
webapp-958cf5567-h247n 0/1 CrashLoopBackOff 3 56s
If the restartPolicy
is set to Always
(default) in the yaml file, the application will continue to restart the pod with an exponential back-off delay in case of failure.
Using InitContaniner
To avoid such a situation, InitContainers
can be defined, which are executed prior to the application container. If one
of the InitContainers
fails, the application container won’t be triggered.
apiVersion: apps/v1
kind: Deployment
metadata:
name: webapp
spec:
selector:
matchLabels:
app: webapp
template:
metadata:
labels:
app: webapp
spec:
initContainers: # check if DB is ready, and only continue when true
- name: check-db-ready
image: postgres:9.6.5
command: ['sh', '-c', 'until pg_isready -h postgres -p 5432; do echo waiting for database; sleep 2; done;']
containers:
- image: xcoulon/go-url-shortener:0.1.0
name: go-url-shortener
env:
- name: POSTGRES_HOST
value: postgres
- name: POSTGRES_PORT
value: "5432"
- name: POSTGRES_DATABASE
value: url_shortener_db
- name: POSTGRES_USER
value: user
- name: POSTGRES_PASSWORD
value: mysecretpassword
ports:
- containerPort: 8080
In the above example, the InitContainers
use the docker image postgres:9.6.5
, which is different from the application container.
This also brings the advantage of not having to include unnecessary tools (e.g. pg_isready) in the application container.
With introduction of InitContainers
, in case the database is not available yet, the pod startup will look like similarly to:
$ kubectl get po -w
NAME READY STATUS RESTARTS AGE
nginx-deployment-5cc79d6bfd-t9n8h 1/1 Running 0 5d
privileged-pod 1/1 Running 0 4d
webapp-fdcb49cbc-4gs4n 0/1 Pending 0 0s
webapp-fdcb49cbc-4gs4n 0/1 Pending 0 0s
webapp-fdcb49cbc-4gs4n 0/1 Init:0/1 0 0s
webapp-fdcb49cbc-4gs4n 0/1 Init:0/1 0 1s
$ kubectl logs webapp-fdcb49cbc-4gs4n
Error from server (BadRequest): container "go-url-shortener" in pod "webapp-fdcb49cbc-4gs4n" is waiting to start: PodInitializing
14 - Out-Dated HTML and JS Files Delivered
Problem
After updating your HTML and JavaScript sources in your web application, the Kubernetes cluster delivers outdated versions - why?
Overview
By default, Kubernetes service pods are not accessible from the external network, but only from other pods within the same Kubernetes cluster.
The Gardener cluster has a built-in configuration for HTTP load balancing called Ingress, defining rules for external connectivity to Kubernetes services. Users who want external access to their Kubernetes services create an ingress resource that defines rules, including the URI path, backing service name, and other information. The Ingress controller can then automatically program a frontend load balancer to enable Ingress configuration.
Example Ingress Configuration
apiVersion: networking.k8s.io/v1beta1
kind: Ingress
metadata:
name: vuejs-ingress
spec:
rules:
- host: test.ingress.<GARDENER-CLUSTER>.<GARDENER-PROJECT>.shoot.canary.k8s-hana.ondemand.com
http:
paths:
- backend:
serviceName: vuejs-svc
servicePort: 8080
where:
- <GARDENER-CLUSTER>: The cluster name in the Gardener
- <GARDENER-PROJECT>: You project name in the Gardener
Diagnosing the Problem
The ingress controller we are using is NGINX. NGINX is a software load balancer, web server, and content cache built on top of open source NGINX.
NGINX caches the content as specified in the HTTP header. If the HTTP header is missing, it is assumed that the cache is forever and NGINX never updates the content in the stupidest case.
Solution
In general, you can avoid this pitfall with one of the solutions below:
- Use a cache buster + HTTP-Cache-Control (prefered)
- Use HTTP-Cache-Control with a lower retention period
- Disable the caching in the ingress (just for dev purposes)
Learning how to set the HTTP header or setup a cache buster is left to you, as an exercise for your web framework (e.g. Express/NodeJS, SpringBoot, …)
Here is an example on how to disable the cache control for your ingress, done with an annotation in your ingress YAML (during development).
---
apiVersion: networking.k8s.io/v1beta1
kind: Ingress
metadata:
annotations:
ingress.kubernetes.io/cache-enable: "false"
name: vuejs-ingress
spec:
rules:
- host: test.ingress.<GARDENER-CLUSTER>.<GARDENER-PROJECT>.shoot.canary.k8s-hana.ondemand.com
http:
paths:
- backend:
serviceName: vuejs-svc
servicePort: 8080
15 - Remove Committed Secrets in Github 💀
Overview
If you commit sensitive data, such as a kubeconfig.yaml
or SSH key
into a Git repository, you can remove it from
the history. To entirely remove unwanted files from a repository’s history you can use the git filter-branch
command.
The git filter-branch
command rewrites your repository’s history, which changes the SHAs for existing commits that you alter and any dependent commits. Changed commit SHAs may affect open pull requests in your repository. Merging or closing all open pull requests before removing files from your repository is recommended.
Warning
If someone has already checked out the repository, then of course they have the secret on their computer. So ALWAYS revoke the OAuthToken/Password or whatever it was immediately.Purging a File from Your Repository’s History
Warning
If you rungit filter-branch
after stashing changes, you won’t be able to retrieve your changes with other
stash commands. Before running git filter-branch
, we recommend unstashing any changes you’ve made. To unstash the
last set of changes you’ve stashed, run git stash show -p | git apply -R
. For more information, see Git Tools - Stashing and Cleaning.To illustrate how git filter-branch
works, we’ll show you how to remove your file with sensitive data from the
history of your repository and add it to .gitignore to ensure that it is not accidentally re-committed.
1. Navigate into the repository’s working directory:
cd YOUR-REPOSITORY
2. Run the following command, replacing PATH-TO-YOUR-FILE-WITH-SENSITIVE-DATA
with the path to the file you want to remove,
not just its filename.
These arguments will:
- Force Git to process, but not check out, the entire history of every branch and tag
- Remove the specified file, as well as any empty commits generated as a result
- Overwrite your existing tags
git filter-branch --force --index-filter \
'git rm --cached --ignore-unmatch PATH-TO-YOUR-FILE-WITH-SENSITIVE-DATA' \
--prune-empty --tag-name-filter cat -- --all
3. Add your file with sensitive data to .gitignore
to ensure that you don’t accidentally commit it again:
echo "YOUR-FILE-WITH-SENSITIVE-DATA" >> .gitignore
Double-check that you’ve removed everything you wanted to from your repository’s history, and that all of your branches are checked out. Once you’re happy with the state of your repository, continue to the next step.
4. Force-push your local changes to overwrite your GitHub repository, as well as all the branches you’ve pushed up:
git push origin --force --all
4. In order to remove the sensitive file from your tagged releases, you’ll also need to force-push against your Git tags:
git push origin --force --tags
Warning
Tell your collaborators to rebase, not merge, any branches they created off of your old (tainted) repository history. One merge commit could reintroduce some or all of the tainted history that you just went to the trouble of purging.Related Links
16 - Using Prometheus and Grafana to Monitor K8s
Disclaimer
This post is meant to give a basic end-to-end description for deploying and using Prometheus and Grafana. Both applications offer a wide range of flexibility, which needs to be considered in case you have specific requirements. Such advanced details are not in the scope of this topic.
Introduction
Prometheus is an open-source systems monitoring and alerting toolkit for recording numeric time series. It fits both machine-centric monitoring as well as monitoring of highly dynamic service-oriented architectures. In a world of microservices, its support for multi-dimensional data collection and querying is a particular strength.
Prometheus is the second hosted project to graduate within CNCF.
The following characteristics make Prometheus a good match for monitoring Kubernetes clusters:
Pull-based Monitoring Prometheus is a pull-based monitoring system, which means that the Prometheus server dynamically discovers and pulls metrics from your services running in Kubernetes.
Labels Prometheus and Kubernetes share the same label (key-value) concept that can be used to select objects in the system.
Labels are used to identify time series and sets of label matchers can be used in the query language (PromQL) to select the time series to be aggregated.Exporters
There are many exporters available, which enable integration of databases or even other monitoring systems not already providing a way to export metrics to Prometheus. One prominent exporter is the so called node-exporter, which allows to monitor hardware and OS related metrics of Unix systems.Powerful Query Language The Prometheus query language PromQL lets the user select and aggregate time series data in real time. Results can either be shown as a graph, viewed as tabular data in the Prometheus expression browser, or consumed by external systems via the HTTP API.
Find query examples on Prometheus Query Examples.
One very popular open-source visualization tool not only for Prometheus is Grafana. Grafana is a metric analytics and visualization suite. It is popular for visualizing time series data for infrastructure and application analytics but many use it in other domains including industrial sensors, home automation, weather, and process control. For more information, see the Grafana Documentation.
Grafana accesses data via Data Sources. The continuously growing list of supported backends includes Prometheus.
Dashboards are created by combining panels, e.g., Graph and Dashlist.
In this example, we describe an End-To-End scenario including the deployment of Prometheus and a basic monitoring configuration as the one provided for Kubernetes clusters created by Gardener.
If you miss elements on the Prometheus web page when accessing it via its service URL https://<your K8s FQN>/api/v1/namespaces/<your-prometheus-namespace>/services/prometheus-prometheus-server:80/proxy
, this is probably caused by a Prometheus issue - #1583. To workaround this issue, set up a port forward kubectl port-forward -n <your-prometheus-namespace> <prometheus-pod> 9090:9090
on your client and access the Prometheus UI from there with your locally installed web browser. This issue is not relevant in case you use the service type LoadBalancer
.
Preparation
The deployment of Prometheus and Grafana is based on Helm charts.
Make sure to implement the Helm settings before deploying the Helm charts.
The Kubernetes clusters provided by Gardener use role based access control (RBAC). To authorize the Prometheus node-exporter to access hardware and OS relevant metrics of your cluster’s worker nodes, specific artifacts need to be deployed.
Bind the Prometheus service account to the garden.sapcloud.io:monitoring:prometheus
cluster role by running the command
kubectl apply -f crbinding.yaml
.
Content of crbinding.yaml
apiVersion: rbac.authorization.k8s.io/v1beta1
kind: ClusterRoleBinding
metadata:
name: <your-prometheus-name>-server
roleRef:
apiGroup: rbac.authorization.k8s.io
kind: ClusterRole
name: garden.sapcloud.io:monitoring:prometheus
subjects:
- kind: ServiceAccount
name: <your-prometheus-name>-server
namespace: <your-prometheus-namespace>
Deployment of Prometheus and Grafana
Only minor changes are needed to deploy Prometheus and Grafana based on Helm charts.
Copy the following configuration into a file called values.yaml
and deploy Prometheus:
helm install <your-prometheus-name> --namespace <your-prometheus-namespace> stable/prometheus -f values.yaml
Typically, Prometheus and Grafana are deployed into the same namespace. There is no technical reason behind this, so feel free to choose different namespaces.
Content of values.yaml
for Prometheus:
rbac:
create: false # Already created in Preparation step
nodeExporter:
enabled: false # The node-exporter is already deployed by default
server:
global:
scrape_interval: 30s
scrape_timeout: 30s
serverFiles:
prometheus.yml:
rule_files:
- /etc/config/rules
- /etc/config/alerts
scrape_configs:
- job_name: 'kube-kubelet'
honor_labels: false
scheme: https
tls_config:
# This is needed because the kubelets' certificates are not generated
# for a specific pod IP
insecure_skip_verify: true
bearer_token_file: /var/run/secrets/kubernetes.io/serviceaccount/token
kubernetes_sd_configs:
- role: node
relabel_configs:
- target_label: __metrics_path__
replacement: /metrics
- source_labels: [__meta_kubernetes_node_address_InternalIP]
target_label: instance
- action: labelmap
regex: __meta_kubernetes_node_label_(.+)
- job_name: 'kube-kubelet-cadvisor'
honor_labels: false
scheme: https
tls_config:
# This is needed because the kubelets' certificates are not generated
# for a specific pod IP
insecure_skip_verify: true
bearer_token_file: /var/run/secrets/kubernetes.io/serviceaccount/token
kubernetes_sd_configs:
- role: node
relabel_configs:
- target_label: __metrics_path__
replacement: /metrics/cadvisor
- source_labels: [__meta_kubernetes_node_address_InternalIP]
target_label: instance
- action: labelmap
regex: __meta_kubernetes_node_label_(.+)
# Example scrape config for probing services via the Blackbox Exporter.
#
# Relabelling allows to configure the actual service scrape endpoint using the following annotations:
#
# * `prometheus.io/probe`: Only probe services that have a value of `true`
- job_name: 'kubernetes-services'
metrics_path: /probe
params:
module: [http_2xx]
kubernetes_sd_configs:
- role: service
relabel_configs:
- source_labels: [__meta_kubernetes_service_annotation_prometheus_io_probe]
action: keep
regex: true
- source_labels: [__address__]
target_label: __param_target
- target_label: __address__
replacement: blackbox
- source_labels: [__param_target]
target_label: instance
- action: labelmap
regex: __meta_kubernetes_service_label_(.+)
- source_labels: [__meta_kubernetes_namespace]
target_label: kubernetes_namespace
- source_labels: [__meta_kubernetes_service_name]
target_label: kubernetes_name
# Example scrape config for pods
#
# Relabelling allows to configure the actual service scrape endpoint using the following annotations:
#
# * `prometheus.io/scrape`: Only scrape pods that have a value of `true`
# * `prometheus.io/path`: If the metrics path is not `/metrics` override this.
# * `prometheus.io/port`: Scrape the pod on the indicated port instead of the default of `9102`.
- job_name: 'kubernetes-pods'
kubernetes_sd_configs:
- role: pod
relabel_configs:
- source_labels: [__meta_kubernetes_pod_annotation_prometheus_io_scrape]
action: keep
regex: true
- source_labels: [__meta_kubernetes_pod_annotation_prometheus_io_path]
action: replace
target_label: __metrics_path__
regex: (.+)
- source_labels: [__address__, __meta_kubernetes_pod_annotation_prometheus_io_port]
action: replace
regex: (.+):(?:\d+);(\d+)
replacement: ${1}:${2}
target_label: __address__
- action: labelmap
regex: __meta_kubernetes_pod_label_(.+)
- source_labels: [__meta_kubernetes_namespace]
action: replace
target_label: kubernetes_namespace
- source_labels: [__meta_kubernetes_pod_name]
action: replace
target_label: kubernetes_pod_name
# Scrape config for service endpoints.
#
# The relabeling allows the actual service scrape endpoint to be configured
# via the following annotations:
#
# * `prometheus.io/scrape`: Only scrape services that have a value of `true`
# * `prometheus.io/scheme`: If the metrics endpoint is secured then you will need
# to set this to `https` & most likely set the `tls_config` of the scrape config.
# * `prometheus.io/path`: If the metrics path is not `/metrics` override this.
# * `prometheus.io/port`: If the metrics are exposed on a different port to the
# service then set this appropriately.
- job_name: 'kubernetes-service-endpoints'
kubernetes_sd_configs:
- role: endpoints
relabel_configs:
- source_labels: [__meta_kubernetes_service_annotation_prometheus_io_scrape]
action: keep
regex: true
- source_labels: [__meta_kubernetes_service_annotation_prometheus_io_scheme]
action: replace
target_label: __scheme__
regex: (https?)
- source_labels: [__meta_kubernetes_service_annotation_prometheus_io_path]
action: replace
target_label: __metrics_path__
regex: (.+)
- source_labels: [__address__, __meta_kubernetes_service_annotation_prometheus_io_port]
action: replace
target_label: __address__
regex: (.+)(?::\d+);(\d+)
replacement: $1:$2
- action: labelmap
regex: __meta_kubernetes_service_label_(.+)
- source_labels: [__meta_kubernetes_namespace]
action: replace
target_label: kubernetes_namespace
- source_labels: [__meta_kubernetes_service_name]
action: replace
target_label: kubernetes_name # Add your additional configuration here...
Next, deploy Grafana. Since the deployment in this post is based on the Helm default values, the settings below are set explicitly in case the default changed.
Deploy Grafana via helm install grafana --namespace <your-prometheus-namespace> stable/grafana -f values.yaml
. Here, the same namespace is chosen for Prometheus and for Grafana.
Content of values.yaml
for Grafana:
server:
ingress:
enabled: false
service:
type: ClusterIP
Check the running state of the pods on the Kubernetes Dashboard or by running kubectl get pods -n <your-prometheus-namespace>
. In case of errors, check the log files of the pod(s) in question.
The text output of Helm after the deployment of Prometheus and Grafana contains very useful information, e.g., the user and password of the Grafana Admin user. The credentials are stored as secrets in the namespace <your-prometheus-namespace>
and could be decoded via kubectl get secret --namespace <my-grafana-namespace> grafana -o jsonpath="{.data.admin-password}" | base64 --decode ; echo
.
Basic Functional Tests
To access the web UI of both applications, use port forwarding of port 9090.
Setup port forwarding for port 9090:
kubectl port-forward -n <your-prometheus-namespace> <your-prometheus-server-pod> 9090:9090
Open http://localhost:9090
in your web browser. Select Graph from the top tab and enter the following expressing to show the overall CPU usage for a server (see Prometheus Query Examples):
100 * (1 - avg by(instance)(irate(node_cpu{mode='idle'}[5m])))
This should show some data in a graph.
To show the same data in Grafana setup port forwarding for port 3000 for the Grafana pod and open the Grafana Web UI by opening http://localhost:3000
in a browser. Enter the credentials of the admin user.
Next, you need to enter the server name of your Prometheus deployment. This name is shown directly after the installation via helm.
Run
helm status <your-prometheus-name>
to find this name. Below, this server name is referenced by <your-prometheus-server-name>
.
First, you need to add your Prometheus server as data source:
- Navigate to Dashboards → Data Sources
- Choose Add data source
- Enter:
Name:<your-prometheus-datasource-name>
Type: Prometheus
URL:http://<your-prometheus-server-name>
Access:proxy
- Choose Save & Test
In case of failure, check the Prometheus URL in the Kubernetes Dashboard.
To add a Graph follow these steps:
- In the left corner, select Dashboards → New to create a new dashboard
- Select Graph to create a new graph
- Next, select the Panel Title → Edit
- Select your Prometheus Data Source in the drop down list
- Enter the expression
100 * (1 - avg by(instance)(irate(node_cpu{mode='idle'}[5m])))
in the entry field A - Select the floppy disk symbol (Save) on top
Now you should have a very basic Prometheus and Grafana setup for your Kubernetes cluster.
As a next step you can implement monitoring for your applications by implementing the Prometheus client API.